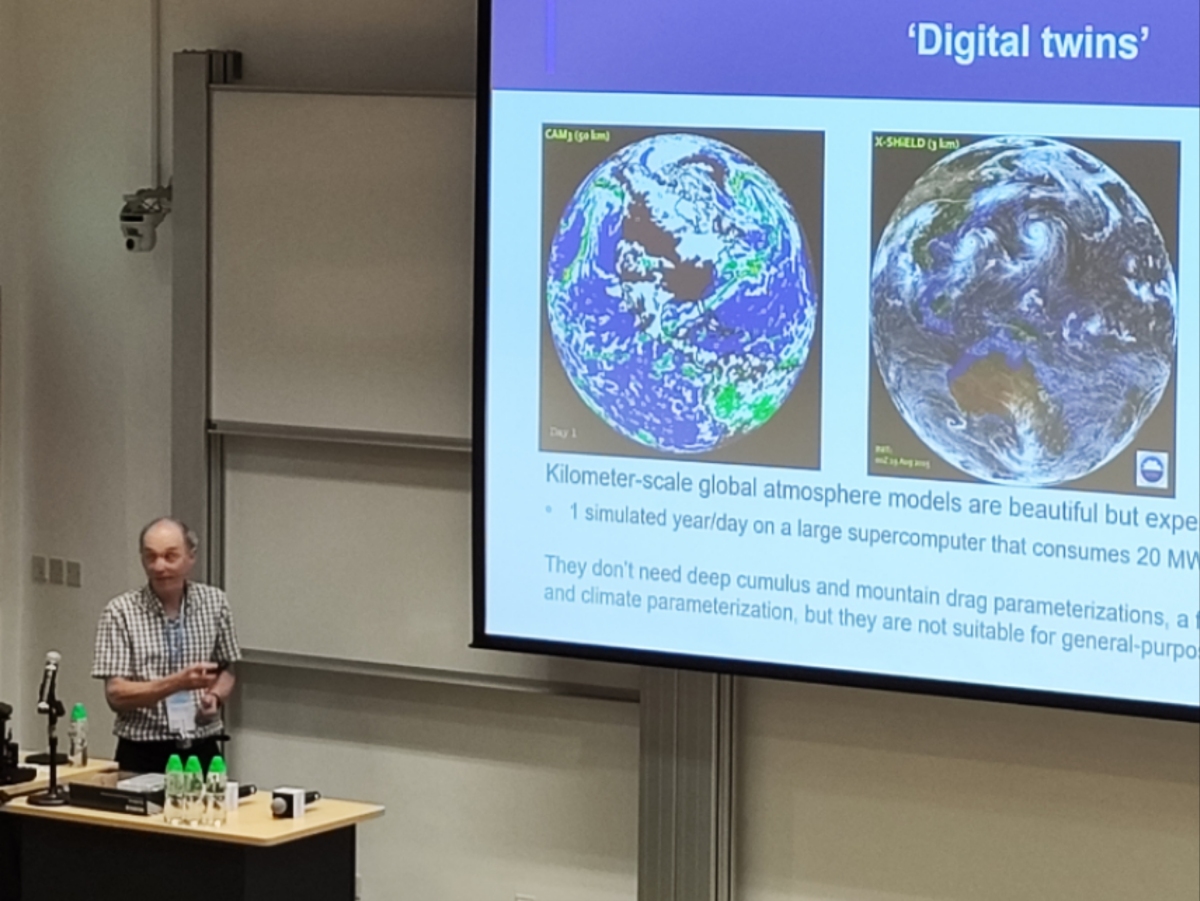
The "Revolutionizing Weather Forecast and Climate Prediction Through Artificial Intelligence" workshop was held today (Sept. 19) at the Hong Kong University of Science and Technology.
During the afternoon session, atmospheric scientist Prof. Christopher Bretherton from the Allen Institute for AI shared insights on AI-based climate emulators, discussing AI's capabilities, challenges, and opportunities in climate modeling.
He highlighted that physically-based climate models have limitations, such as systematic errors and the high cost of running the simulations, while machine learning has the potential to improve climate modeling by reducing systematic biases and enhancing efficiency. Machine learning can be employed to correct physical parameterizations in climate models.
One thing machine learning is very good at doing is to reduce systematic biases, said Prof. Bretherton. "If you have a training data set, machine learning will tend to create an object capable of making predictions, as long as you stay close to that training data set that does not have biases."
He also mentioned two main strategies in weather and climate machine learning models: hybrid machine learning and full model emulation. Full model emulation, where the entire model is replaced by machine learning, can run efficiently on GPUs and extend simulation time beyond conventional models. "It's not limited by standard numerical analysis stability limits, it makes full model emulation about 100 times faster than running a conventional model with the same number of critical points," he added.
Prof. Bretherton explained that machine learning models for weather are typically trained to produce accurate short-term forecasts, often within a range of six to 24 hours. Some models have also shown promising results in predicting weather conditions up to three days ahead.
Addressing the challenges, he noted the pitfalls of hybrid machine learning, including feedback loops between machine learning components and physical processes. Therefore, careful selection of inputs and outputs is crucial.
He also mentioned the limitations of machine learning models in extrapolation and their unreliability in certain applications, as well as the challenges in capturing the full complexity of climate systems, suggesting that these models may not be suitable for scientists who need to understand model sensitivity to specific parameters.
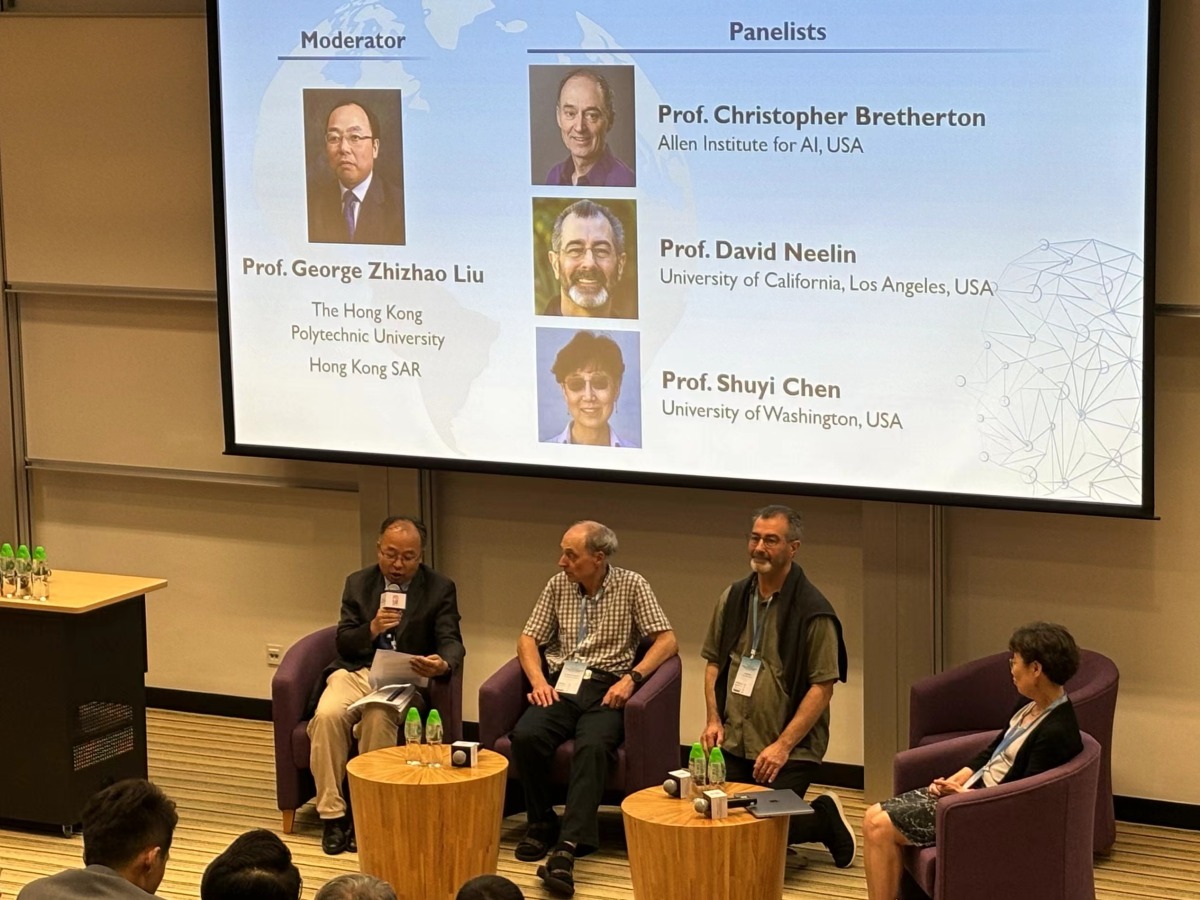
During the panel discussion, Prof. David Neelin from the University of California and Prof. Chen Shuyi from the University of Washington joined Prof. Bretherton to address questions of interest from attendees.
Prof. Neelin shared insights from his ocean science and climate change research, particularly regarding how to integrate AI models with physical numerical models, mentioning the connections between Fortran, Python, and C++ for model conversion.
Prof. Chen discussed her research on extreme weather and hurricane-ocean interactions, emphasizing the importance of validating multi-scale processes from weather to climate, and highlighted how AI and machine learning can help bridge the gap between the two.
Related news:
Experts: AI models in weather nowcasting being helpful and competitive
Comment