Experts: AI models in weather nowcasting being helpful and competitive

AI models are now being very competitive compared with conventional models, and can be helpful in giving really useful data, said experts on today's (Sept. 19) forum themed on Revolutionizing Weather Forecast and Climate Prediction Through AI held at HKUST.
AI models play significant roles in nowcasting
The Hong Kong Observatory (HKO) has already employed different kinds of AI models for weather nowcasting in reality, and machine learning is the way to go, said Dr. Wong Wai-kin, Senior Scientific Officer from HKO.
He highlighted that by using these models, the Observatory has gained greater efficiency and accuracy in predicting weather events such as rainfalls and lightning. By using Generative Adversarial Network (GAN), for example, we will have a lower false alarm compared to optical flow and a higher skill score, but it also has some drawbacks in getting the stable training and has some artifacts generated due to the design of the GAN training network, he pointed out.
The benefits of AI models in providing high-resolution insights, especially in the application of predicting extreme weather is noteworthy. Mr. Wong stressed that the integration of physics-based models with machine learning to enhance forecasting accuracy is important, with experiments showing significant improvements in predicting tropical cyclone intensity and band structures being conducted.
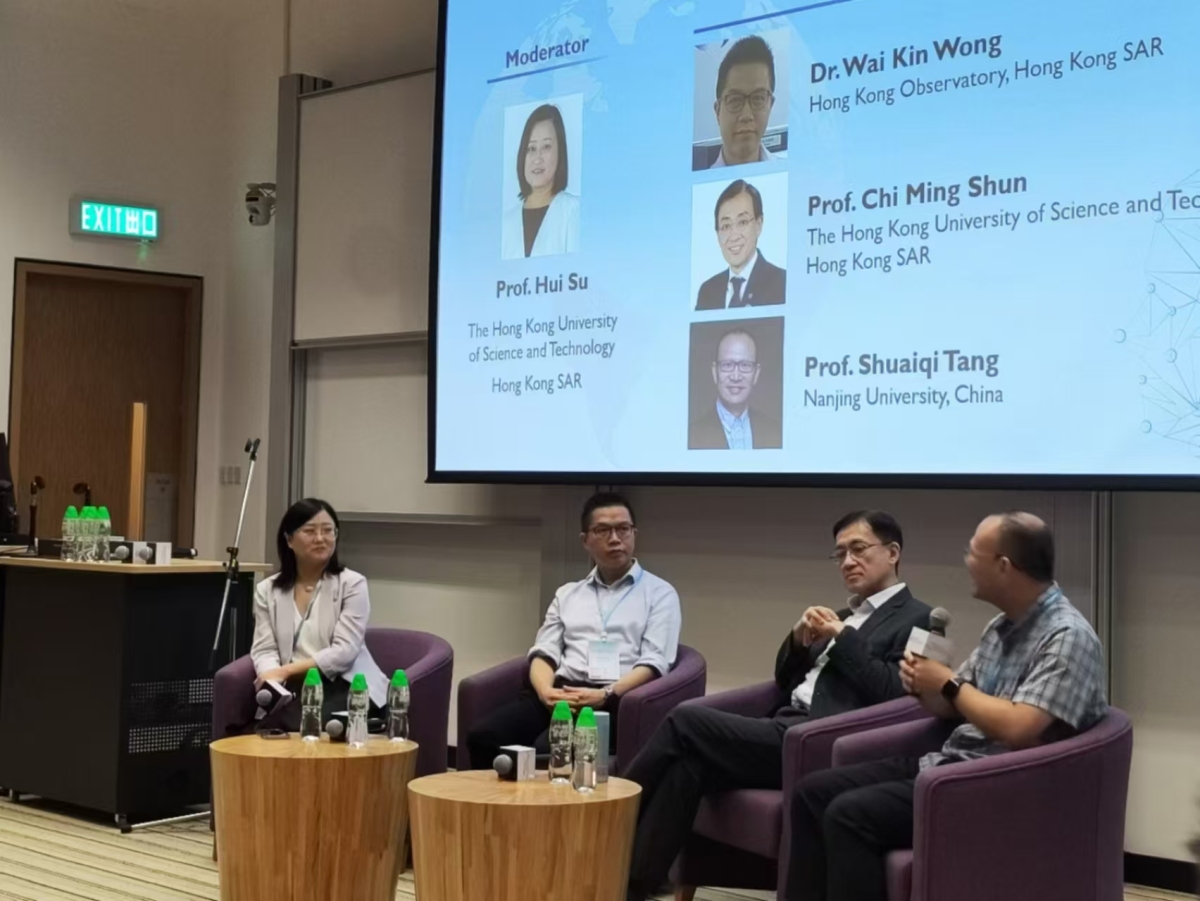
AI models in weather nowcasting offer more opportunities than threats
Although the rapid advancements in AI models by tech companies like Nvidia, Google, and Huawei are now competitive with traditional models, they offer more opportunities than threats. Dr. Wong, speaking from the perspective of weather forecasting, mentioned that the good performance of AI in weather forecasting, such as simulating by representing the trend room, steering flow, and the big patterns of atmosphere, has been verified.
He also stressed the importance of partnerships and the involvement of companies like Google and Microsoft in pilot projects to enhance weather forecasting capabilities.
From a user's standpoint, Prof. Shun Chi-ming from HKUST agreed with Dr. Wong on the superior performance of AI models and noted the early indications of AI models outperforming traditional models in typhoon seasons. He also emphasized the need for explainable AI models for decision-making.
Better weather nowcasting requires data with high quality and large scale, and also comprehensive observation
Data is the foundation for training effective AI models, and different types of data may lead to diverse AI functions. In terms of nowcasting extreme rainfall, data with good quality, large volume, and comprehensive observation are essential.
Data should be sufficient and good enough, and a rain-engaged network is also needed. Although we may not fully understand why AI models can perform so well, these models provide skills in representing large-scale weather phenomena. This, in turn, may help us better understand the background atmospheric conditions and give a more complete picture of why we experience extreme weather, said Dr. Wong.
"I think the size of the data is one critical aspect," Prof. Shun stressed. He also mentioned that human knowledge needs to be embedded in the training datasets. "We need to tell the AI about all these cases and categories, like what a frontal surface is, and what a cold front is, to achieve more accurate rainfall predictions," he said.
"What I feel is that observation is not enough and not complete," said Prof. Tang Shuaiqi from Nanjing University. Although data with good quality and real-time analysis is available, when it comes to severe weather, people heavily rely on radar images. This means that some variables, like cloud structures and even turbulence scales, are not well observed. Improving these areas of observation may be a direction we need to focus on, he added.
Related news:
Comment